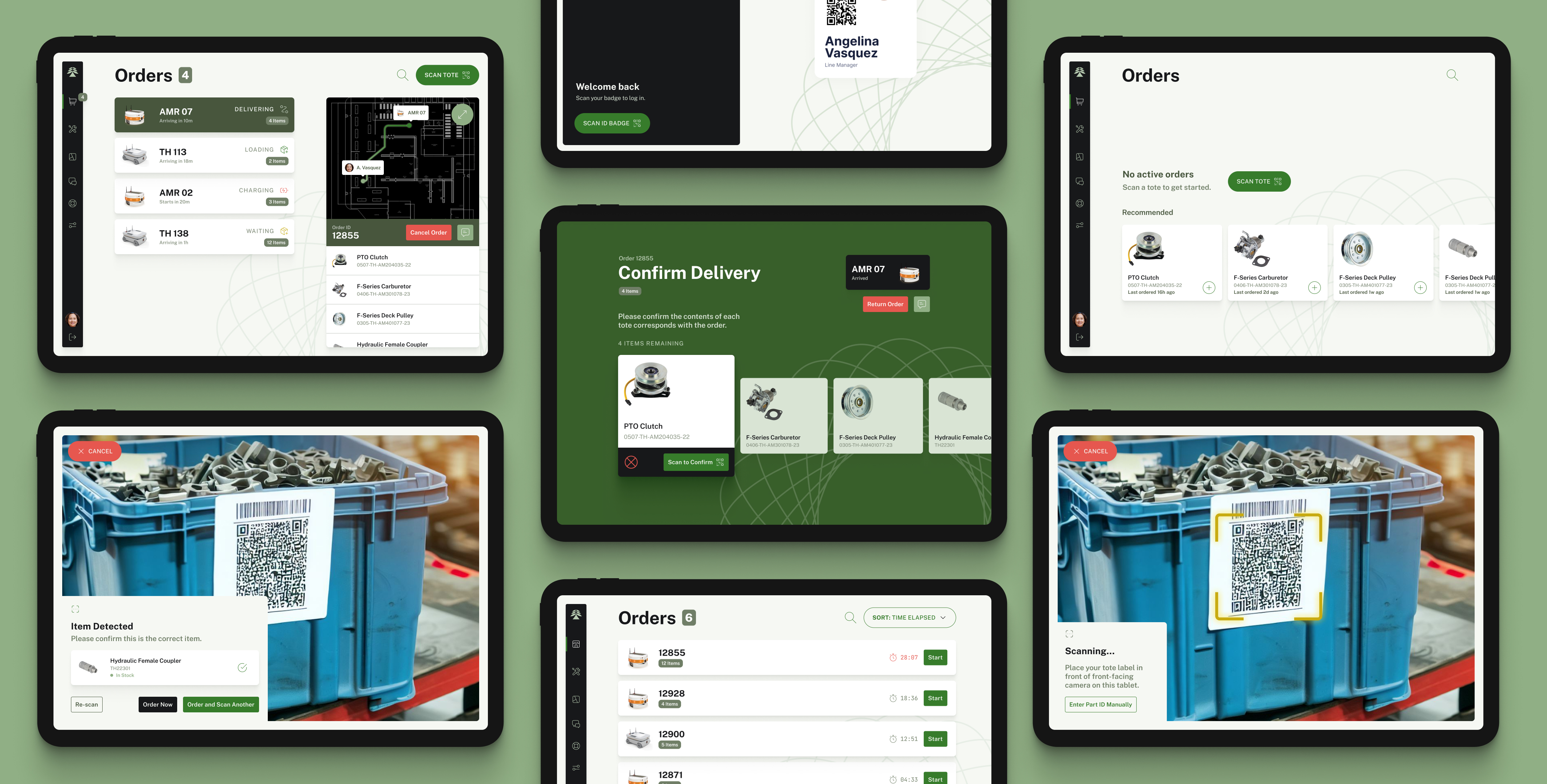
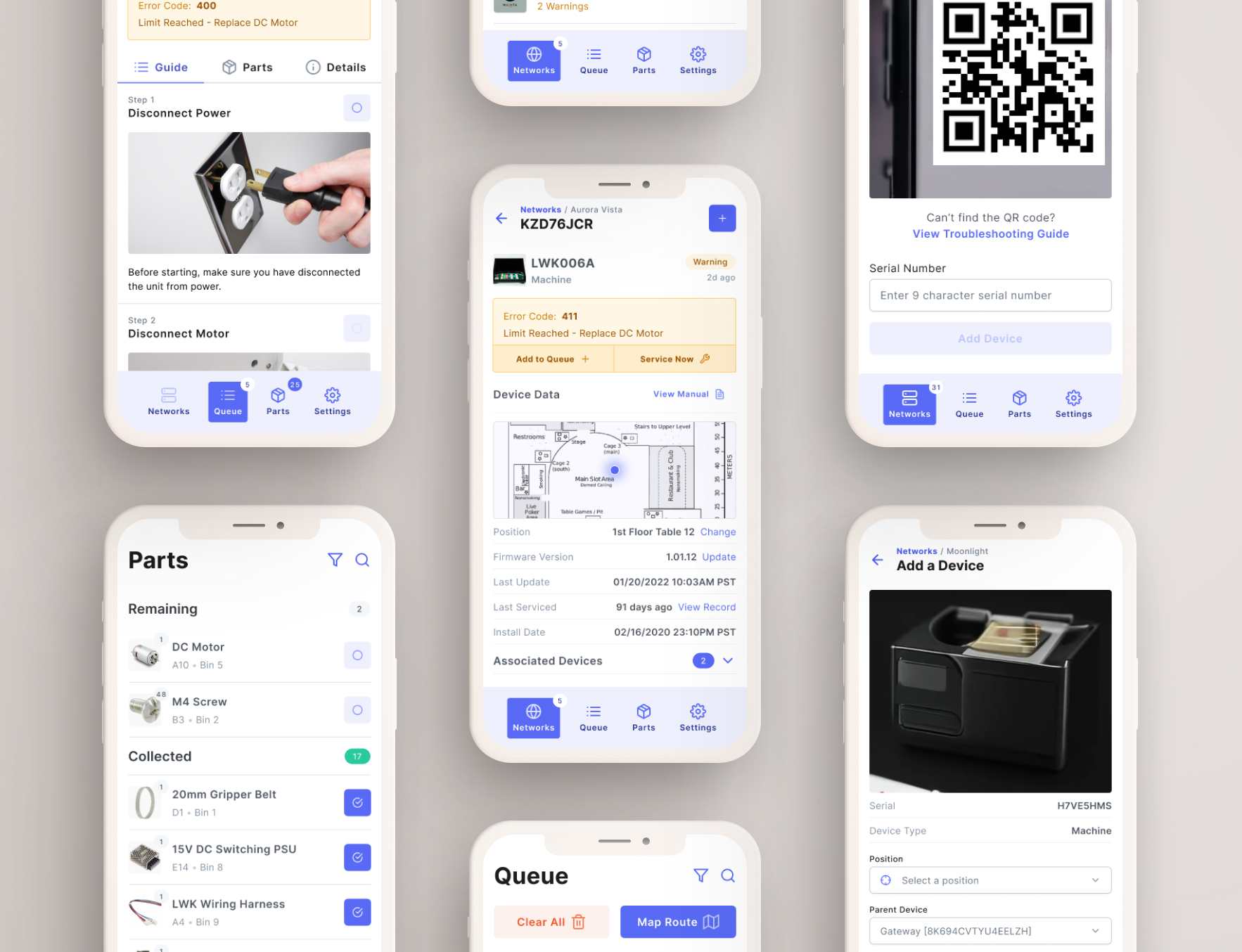
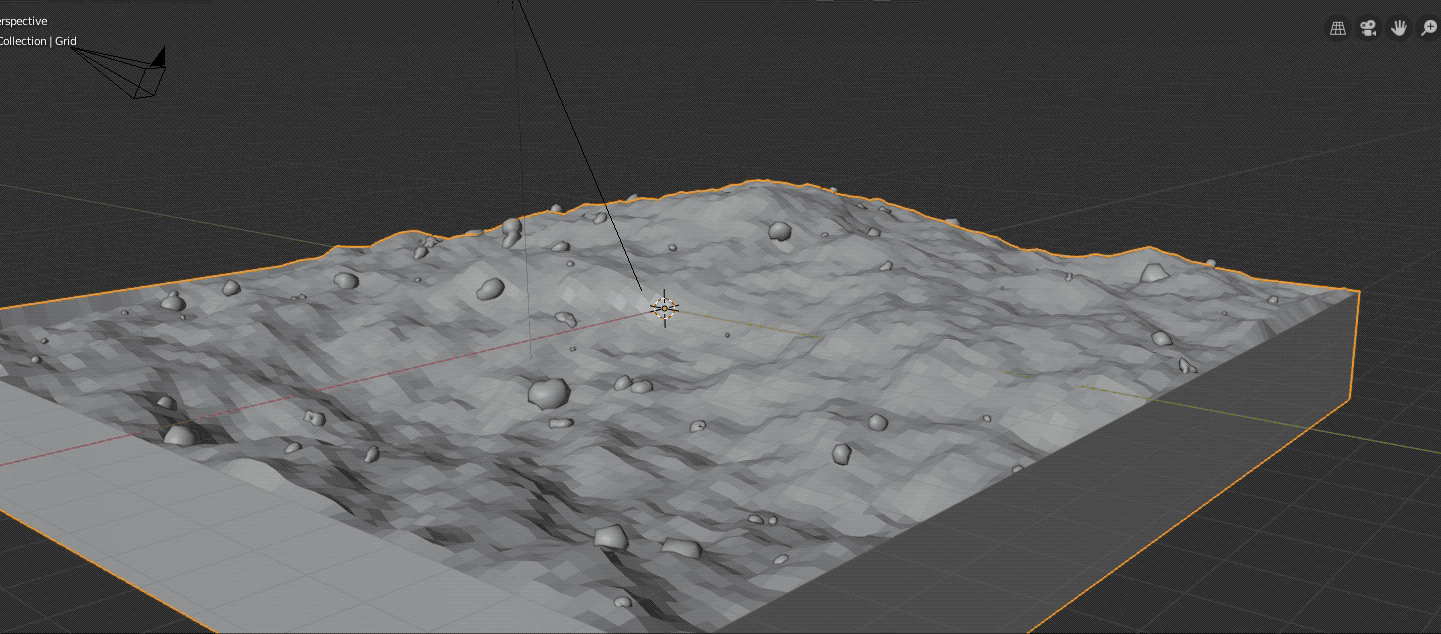
My résumé is best seen on LinkedIn. Otherwise, you can reach me via this contact form. I am currently the Head of Design for PanasonicWELL, a US-based R&D group working at the intersection of connected devices, families, and wellness.
Most recently served as Principal Design Technologist for AWS Prototyping & Cloud Engineering in NYC from 2019 - 2023. I designed and built human-centric prototypes that employ Artificial Intelligence, Machine Learning, Robotics, Internet of Things, and Spatial Computing. Previously a UX Director for start-ups and digital creative advertising.
Most recently served as Principal Design Technologist for AWS Prototyping & Cloud Engineering in NYC from 2019 - 2023. I designed and built human-centric prototypes that employ Artificial Intelligence, Machine Learning, Robotics, Internet of Things, and Spatial Computing. Previously a UX Director for start-ups and digital creative advertising.
Organizations I’ve worked with include:
- NASA/JPL
- Wendy's
- Lloyds of London
- Better.com
- Bridgestone
- The New York Times R&D
- Veeva
- Disney
- John Deere
- PODS
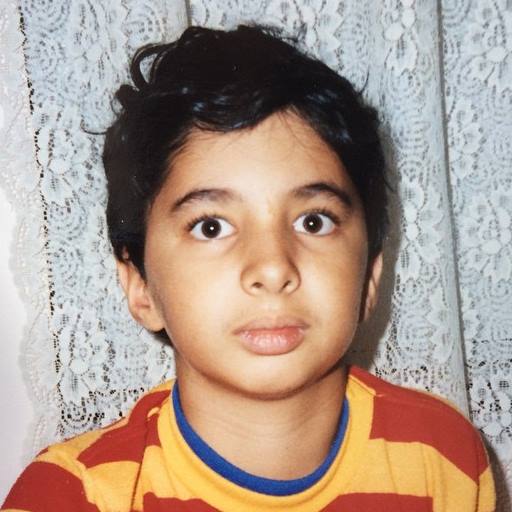
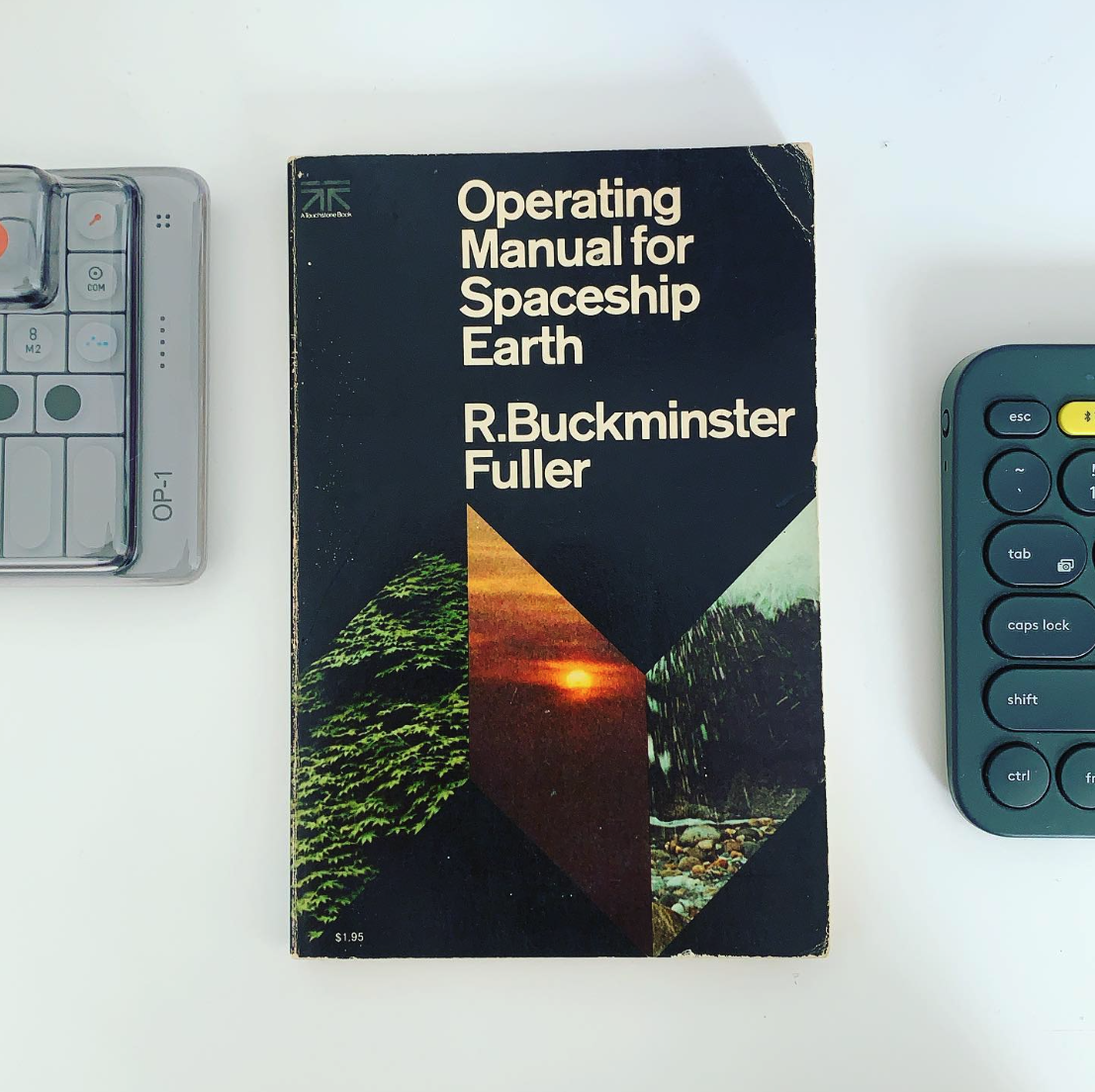
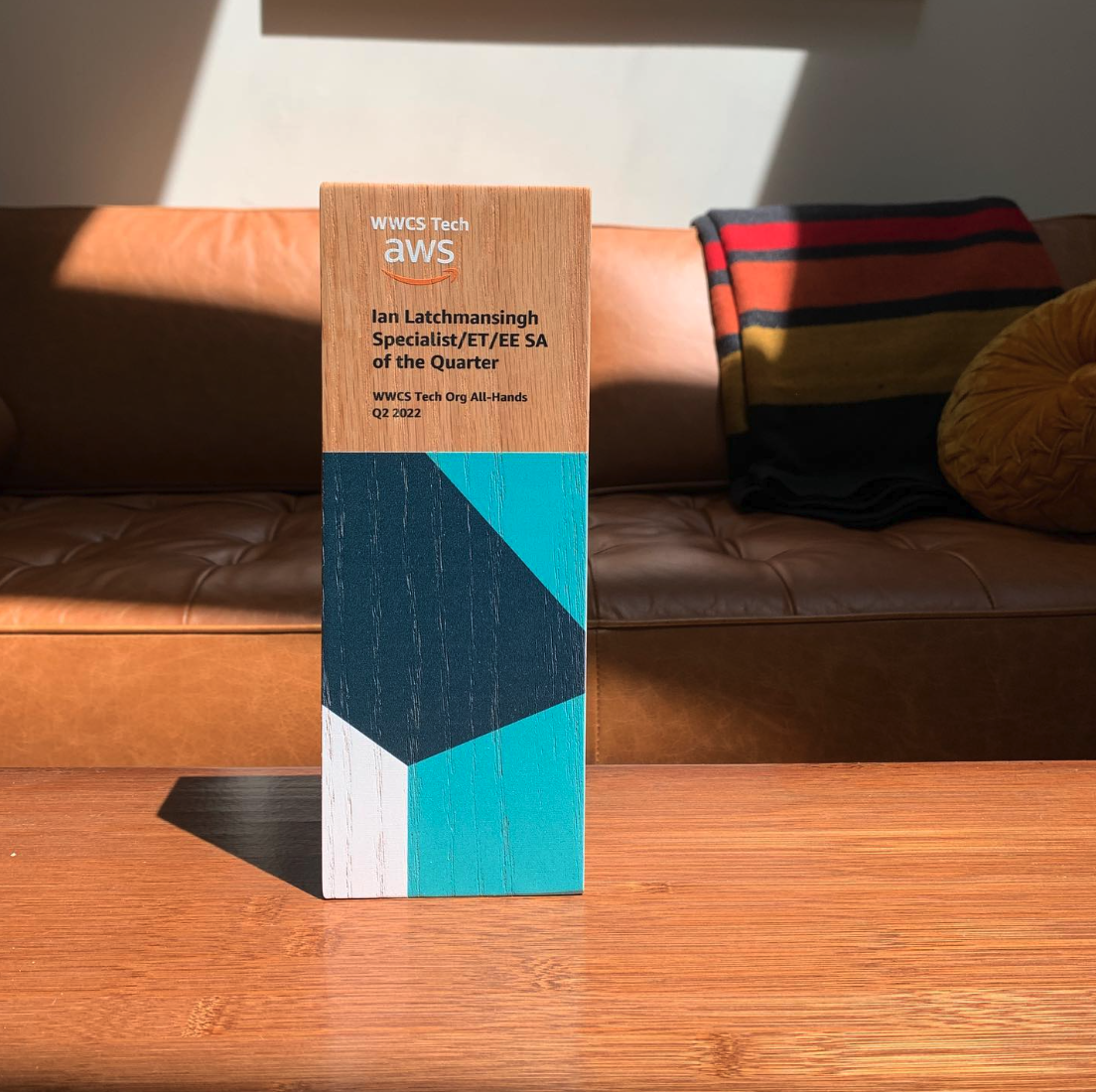
PROTOTYPES & PRODUCTS
Industrial
- Virtual Remote Auditing for GxP Compliance
- CV/ML Detection of Product Defects in Aluminum Manufacturing with Fully-Synthetic Data
- Agricultural Cost Center Business Intelligence for IoT-equipped Farms
- Realtime Inventory Forecasting for Just-in-Time Manufacturing
- Supply Chain Weakness Dashboards for Agricultural Logistics
- Municipal Infrastructure Prediction and Planning Tools
- Disaster Risk Monitoring Search Interfaces for Restoration Contractors
Consumer
- VUIs for Drive Thru Restaurants
- Consumer Care Care Subscription Services
- Live Interactive Journalism
- Virtual Doping Detection for Physical E-Sports
- Online Learning for First-Time Homebuyers
Business
- VUI Design for Clinical Trials and Medication Adherence
- Distributed Consumables Procurement for Container Shipping
- Connected Devices for C-Store Cleanliness Monitoring and Task Management
- Predictive Fleet Vehicle Maintenance for SMBs
- Intelligent Damage Detection for Rental Vehicles
- Hybrid UIs for Realtime Customer Service Analytics
Broadcast Monitoring using Machine Learning and Computer Vision
Broadcast monitoring is a service provided to broadcasters and over-the-top (OTT) streamers that provides a significant number of quality checks on a given media source. They can be relatively minor errors like spelling or audio volume, or more critical issues like content errors (broadcasting the wrong media) and incorrect audio (wrong language or content).
Traditionally, the higher-level quality checks are conducted manually by humans who are constantly watching broadcast streams for issues and escalating them to the sources. An operator may be watching anywhere from six to 34 simultaneous streams, which indicates this is non-scalable with the available workforce. As OTT streams in particular increase, it may become essential for quality monitoring services to augment their workforce with machine learning.
This solution, for which I designed and developed the interface, allows for the automation of higher-level monitoring tasks that were previously manual chores. This enables human workers to focus on higher-level tasks, take action sooner, and handle a higher volume of broadcasts without sacrificing efficacy.
This prototype was developed in six weeks alongside engineers Adam Best and Angela Rouhan Wang, who utilized AWS AI services like Amazon Rekognition to analyze the content of an HTTP Live Streaming (HLS) video stream. This is done in near real-time (sub 15 seconds per sample).
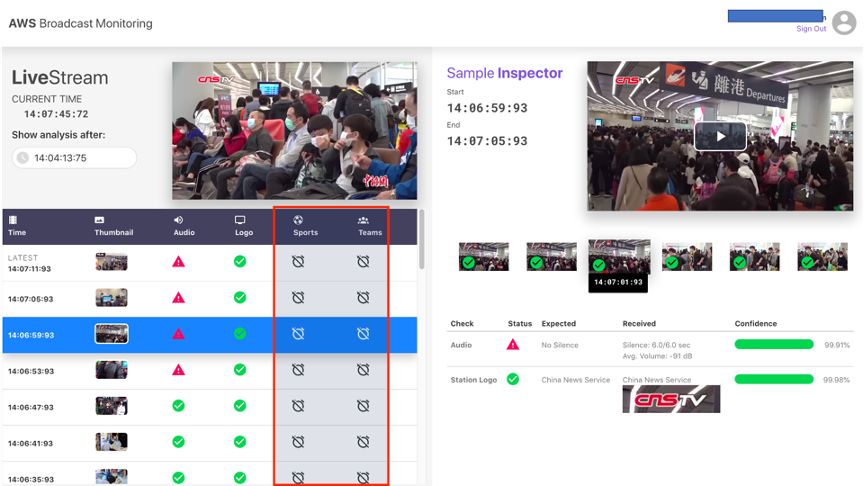
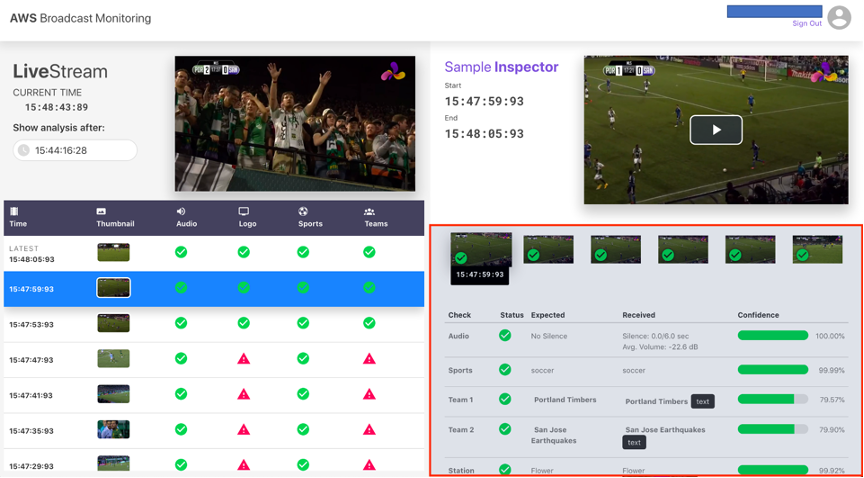
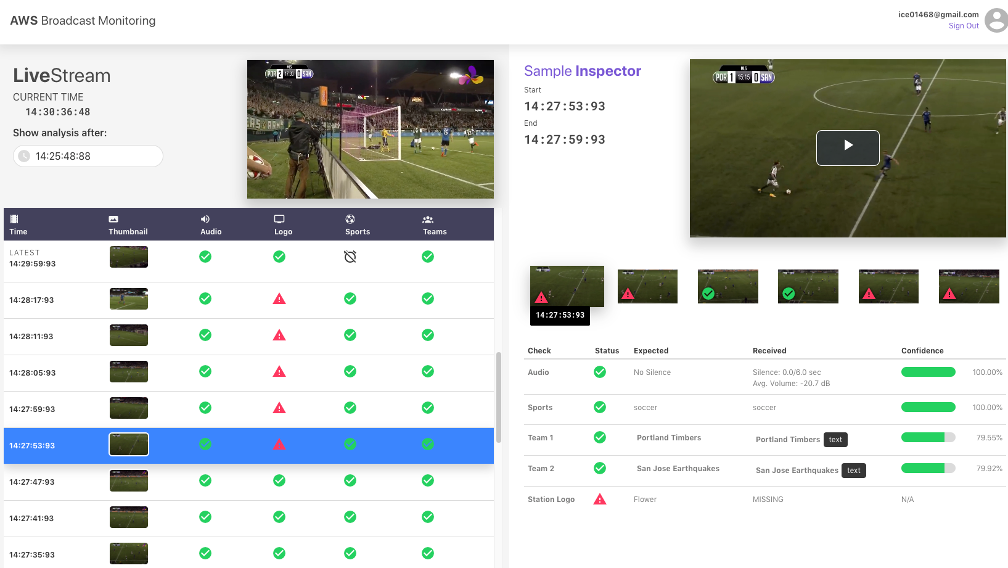
©MMXXI
Design is done when there is nothing left to take away.